Neuromorphic & Bio-inspired Computing
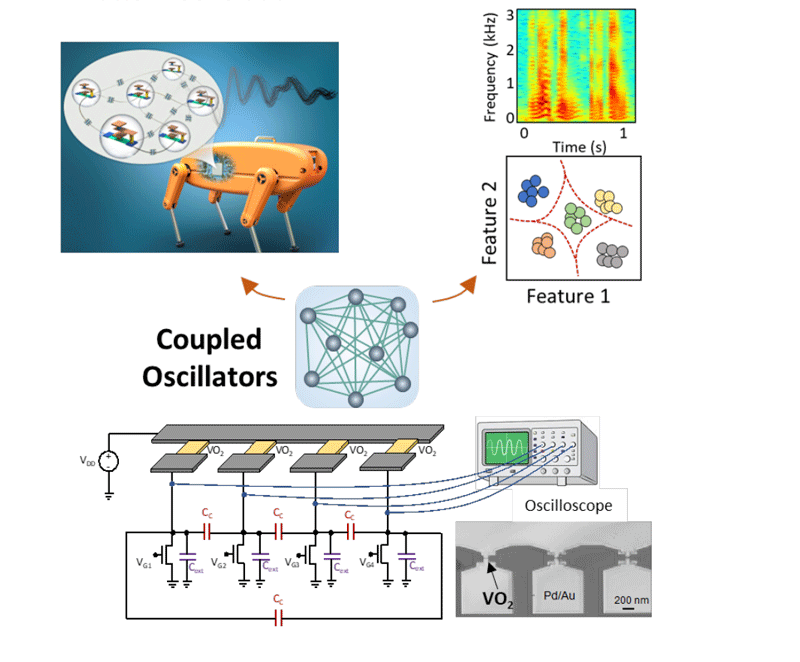
Coupled Oscillator Dynamical Systems
Biologically inspired computing harnesses the emergent phenomena in dynamical systems such as coupled oscillator-based attractor networks for pattern generation as well as pattern classification. In one recent work we show a striking similarity between biological locomotion gaits and the evolution of phase patterns in a coupled oscillatory network to create a central pattern generator. Vanadium Dioxide (VO2) oscillators are fabricated and exhibit stable self-oscillations when connected with biasing transistors. We demonstrate the hardware for low-power compact nano-oscillators that are bi-directionally coupled using capacitors and create an energy-efficient CPG hardware that will function as the neural oscillators do in the body. In another work, we exploit the synchronization dynamics of these coupled oscillators to classify complex temporal patterns and create an ultra-compact and low power classifier for spoken vowel signals.
Papers:
1. Dutta, Sourav, et al. "Programmable coupled oscillators for synchronized locomotion." Nature communications 10.1 (2019): 1-10.
2. Dutta, S., et al. "Spoken vowel classification using synchronization of phase transition nano-oscillators." 2019 Symposium on VLSI Technology. IEEE, 2019.
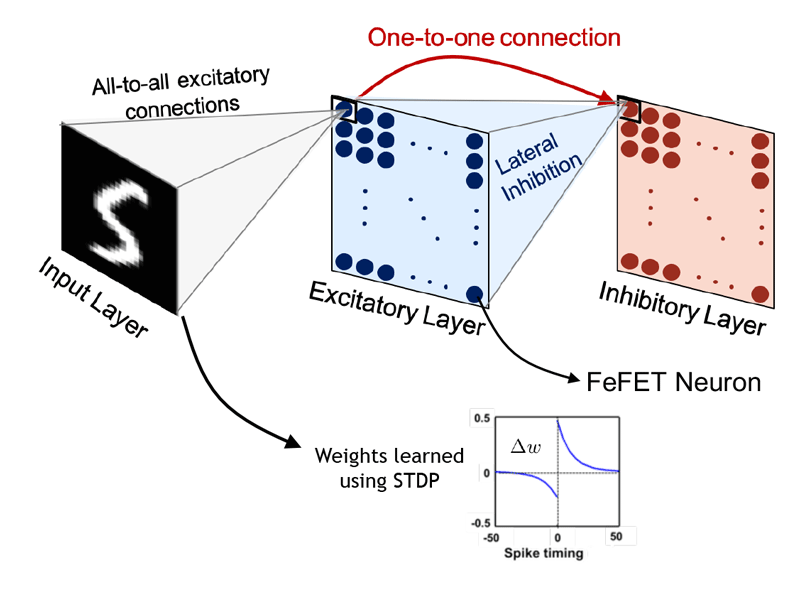
FeFET-based Spiking Neural Network
FeFET-based Spiking Neural Network
The human brain’s cognitive power involves collective computing over an ensemble of imprecise components, aka neurons and synapses operating in a highly parallel fashion. This has spurred researchers to explore the remarkable mechanisms underpinning biological cognitive computing towards replicating the brain on a “neuromorphic chip”. Exploiting the physics of nano-scale devices to emulate the biophysical characteristics of neurons and synapses while treating their intrinsic stochasticity as a resource provides a promising avenue for enabling low-power, dense neuromorphic hardware. We investigate how the intricate dynamics of polarization switching and relaxation in HfZrO2 -based ferroelectric field-effect transistor (FeFET) can be exploited to mimic adaptive, stochastic spiking neurons and synapses.
Papers:
1. Dutta, S., et al. "Biologically Plausible Ferroelectric Quasi-Leaky Integrate and Fire Neuron." 2019 Symposium on VLSI Technology. IEEE, 2019.
Funded by:
NSF, EXCEL, DARPA, SRC